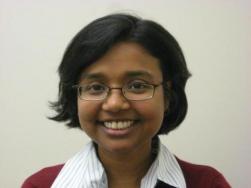
With the proliferation of big data and machine learning algorithms to analyze all of that data, more and more sensitive information about individuals is raising the risk of invading those individuals' privacy. That's the topic -- "Challenges in Privacy-Preserving Data Analysis" -- that CSE Prof. Kamalika Chaudhuri will tackle in a high-profile speech to the 19th International Conference on Artificial Intelligence and Statistics (AISTATS 2016). Chaudhuri is one of only three experts invited to deliver keynote presentations at AISTATS 2016, which will be held in Cadiz, Spain, May 9-11.
AISTATS is an interdisciplinary gathering of researchers at the intersection of computer science, artificial intelligence, machine learning, statistics, and related areas. Chaudhuri (at right) is an expert in the theoretical foundations of machine learning.
In her talk, Chaudhuri will explore how to design data analysis algorithms that operate on the sensitive data of individuals while still guaranteeing the privacy of individuals in the data. She will address two particular challenges to the preservation of privacy. The first problem involves privacy-preserving classification, and a proposed classifer that works "in the ERM (empirical loss minimization) framework, and includes privacy preserving logistic regression and privacy preserving support vector machines." Chaudhuri will also address the question of learning from sensitive correlated data, including private information on users connected together in a social network. Another example, she notes, would involve measurements of physical activity of a single user across time. Chaudhuri explores a new generalization of differential privacy and will prsent new privacy mechanisms in the framework.