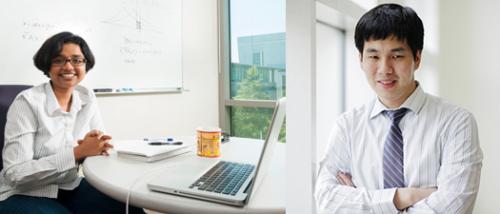
The Bloomberg Data Science Research Grant Program, run by the financial giant created by billionaire former New York City mayor Michael Bloomberg, is a relatively new source of support for computer-science research. It began in 2015. Specifically, faculty can apply for unrestricted gifts to support research in data science, including natural-language processing, machine learning, and data mining. The latest round of grants drew hundreds of applications. Only eight projects were selected for funding, and only six of the winning teams were based at U.S. universities.
Despite the odds, one of the winning teams consisted of computer science professors at Columbia University and UC San Diego -- one a CSE alumnus, the other a former CSE postdoctoral researcher..
CSE Prof. Kamalika Chaudhuri (far left) was a postdoc in Calit2's Information Theory and Applications Center (2007-2009) and in CSE (2009-2010), before joining the department faculty. She teamed with CSE alumnus Daniel Hsu (Ph.D. '10), an assistant professor at Columbia, on a project titled "Spectral Learning with Prior Information with Applications to Topic Models." According to Chaudhuri, the goal of this project is to design algorithms and statistical tools to build complex probabilistic models from massive quantities of data in a computationally-efficient manner. "Recent advances in machine learning have led to the development of spectral learning, an efficient method for learning probabilistic graphical models, that can work with such massive quantities of data," said Chaudhuri. "But existing spectral-learning methods cannot utilize auxiliary information that the modeler may have, which limits their applicability."
In their winning proposal, Hsu -- who did his Ph.D. under CSE Prof. Sanjoy Dasgupta -- and Chaudhuri proposed to address this limitation by designing a framework and algorithms for injecting prior knowledge into spectral learning through constrained optimization.
As outlined by Bloomberg in announcing the winning projects, "Complex statistical models are challenging to fit to large, high-dimensional data sets. Although several recent developments in machine learning have led to scalable fitting methods based on simple algebraic techniques, they are unable to incorporate prior knowledge constraints into the model fitting. Professors Chaudhuri and Hsu will develop new extensions of these scalable methods that can handle such constraints, and they will apply these methods to perform comparative analyses of large document corpora."
Columbia's Hsu is a pioneer of spectral learning for natural-language processing (NLP) applications, and he is PI on the project. Co-PI Chaudhuri has also published on spectral learning, including preliminary work on using constrained spectral learning to compare epigenetic sequences from related cell types. The two computer scientists have co-authored six publications over the past seven years. The $60,000 Bloomberg grant will fund two Ph.D. students (one at UCSD, one at Columbia) for one semester each, in addition to some summer support for Chaudhuri.
Learn more or apply for future grants through The Bloomberg Data Science Research Grant Program.