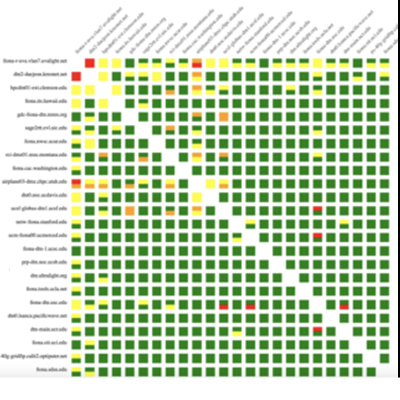
Effective October 1, 2017, CSE Professor and Calit2 Director Larry Smarr will become Principal Investigator on a new NSF-funded, $1 million community infrastructure in support of machine learning research. Ahead of the launch, HPCwire interviewed Smarr for a preview of the proposed cyberinfrastructure, which includes two co-PIs affiliated with CSE: Professor Tajana Rosing, and CSE lecturer Ilkay Altintas, who is also SDSC's Chief Data Science Officer. A much longer list of potential users of the community infrastructure includes CSE professors Ravi Ramamoorthi, Manmohan Chandraker, Arun Kumar, Rajesh Gupta, Gary Cottrell, and others. Following is an excerpt from HPCwire's July 25 article:

The ambitious plan – Cognitive Hardware and Software Ecosystem, Community Infrastructure (CHASE-CI) – is intended to leverage the high-speed Pacific Research Platform (PRP) and put fast GPU appliances into the hands of researchers to tackle machine learning hardware, software, and architecture issues.
Given the abrupt rise of machine learning and its distinct needs versus traditional FLOPS-dominated HPC [High Performance Computing], the CHASE-CI effort seems a natural next step in learning how to harness PRP’s high bandwidth for use with big data projects and machine learning. Perhaps not coincidentally [CSE's] Smarr is also principal investigator for PRP. As described in the NSF abstract, CHASE-CI “will build a cloud of hundreds of affordable Graphics Processing Units (GPUs), networked together with a variety of neural network machines to facilitate development of next generation cognitive computing.”
Those are big goals. Last week, Smarr and co-PI Thomas DeFanti spoke with HPCwire about the CHASE-CI project. It has many facets. Hardware, including von Neumann (vN) and non von Neumann (NvN) architectures, software frameworks (e.g., Caffe and TensorFlow), six specific algorithm families (details near the end of the article), and cost containment are all key target areas. In building out PRP, the effort leveraged existing optical networks such as GLIF by building termination devices based on PCs and providing them to research scientists. The new device — dubbed FIONA (Flexible I/O Network Appliances) – was developed by PRP co-PI Philip Papadopoulos and is critical to the new CHASE-CI effort. A little background on PRP may be helpful.
As explained by Smarr, the basic PRP idea was to experiment with a cyberinfrastructure that was appropriate for a broad set of applications using big data that aren’t appropriate for the commodity internet because of the size of the datasets. To handle the high-speed bandwidth, you need a big bucket at the end of the fiber, notes Smarr. FIONAs filled the bill; the devices are stuffed with high performance, high capacity SSDs and high speed NICs but based on the humble and less expensive PC.

time period ending in April 2017.
“They could take the high data rate without TCP backing up and thereby lowering the overall bandwidth, which traditionally has been a problem if you try to go directly to spinning disk,” says Smarr. Currently, there are on the order of 40 or 50 of these FIONAs deployed across the West Coast. Although 100 gigabit throughput is possible via the fiber, most researchers are getting 10 gigabit, still a big improvement.
DOE tests the PRP performance regularly using a visualization tool MadDash (Monitoring and Debugging Dashboard). “There are test transfers of 10 gigabytes of data, four times a day, among 25 organizations, so that’s roughly about 300 transfers four times a day. The reason why we picked that number, 10 gigabytes, was because that’s the amount of data you need to get TCP up to full speed,” says Smarr.
Networks are currently testing out at 5, 6, 7, 8 and 9 gigabits per second, which is nearly full utilization. “Some of them really nail it at 9.9 gigabits per second. If you go to 40 gigabit networks that we have, we are getting 13 and 14 gigabits per second and that’s because of the [constrained] software we are using. If we go to a different software, which is not what scientists routinely use [except] the high energy physics people, then we can get 30 or 40 or 100 gigabits per second – that’s where we max out with the PC architecture and the disk drives on those high end units,” explains DeFanti.
[Editor's note: To read the full original article, click here to view the complete report on the HPCwire website.]