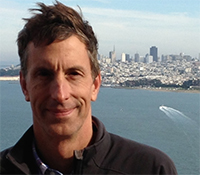
A special seminar in Artificial Intelligence featured a talk Nov. 9 by University of Wisconsin-Madison professor Robert Nowak. He focused on "Learning Human Preferences and Perceptions from Data." CSE Prof. Kamalika Chaudhuri hosted the event.
According to Nowak (pictured at right), modeling human preferences and perceptions has many applications in cognitive, social, and educational science, as well as in advertising and commerce. This talk discussed theory and methods for learning rankings and embeddings representing perceptions from datasets of human judgments, such as ratings or comparisons. Professor Nowak briefly described an ongoing, large-scale experiment with the New Yorker magazine that dealt with ranking cartoon captions using his group's nextml.org system. He also discussed recent work on ordinal embedding, also known as non-metric multidimensional scaling, which is the problem of representing items (e.g., images) as points in a low-dimensional Euclidean space given constraints of the form "item i is closer to item j than item k.” In other words, the goal is to find a geometric representation of data that is faithful to comparative similarity judgments. This classic problem is often used to gauge and visualize perceptual similarities. A variety of algorithms exist for learning metric embeddings from comparison data, but the accuracy and performance of these methods were poorly understood. Nowak presented a new theoretical framework that quantifies the accuracy of learned embeddings and indicated how many comparisons suffice as a function of the number of items and the dimension of the embedding. This theory also points to new algorithms that outperform previously proposed methods. Nowak also described a few applications of ordinal embedding. This joint work with Lalit Jain and Kevin Jamieson. For more information, visit http://nextml.org/assets/next.pdf or https://arxiv.org/pdf/1606.07081v1.pdf.
Robert Nowak is the McFarland-Bascom Professor in Engineering at the University of Wisconsin-Madison, where his research focuses on signal processing, machine learning, optimization, and statistics. Nowak is a professor in Electrical and Computer Engineering, as well as being affiliated with the departments of Computer Sciences, Statistics, and Biomedical Engineering at the University of Wisconsin. He is also a Fellow of the IEEE and the Wisconsin Institute for Discovery.
Nowak is also an Adjunct Professor at the Toyota Technological Institute at Chicago, a member of the Wisconsin Optimization Research Consortium and Machine Learning @ Wisconsin, and organizer of the SILO seminar series.