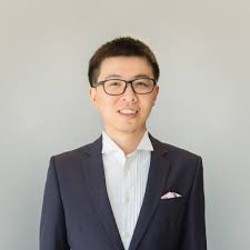
By Josh Baxt
When new UC San Diego Computer Science and Engineering (CSE) Assistant Professor Jingbo Shang was in fifth grade, he entered a computer programming competition in his hometown, his first foray into computer science. He was using Pascal, a computer language co-developed in the 1970s by former UC San Diego Professor Kenneth Bowles. Foreshadowing, perhaps?
Shang earned his bachelor’s degree in computer science at Shanghai Jiao Tong University in 2014 and his Ph.D. at the University of Illinois, Urbana-Champaign in 2019. Later that year, he join the faculty at UC San Diego, with a joint appointment at CSE and the Halıcıoğlu Data Science Institute (HDSI).
“I’m mainly working on data, mining, natural language processing and machine learning,” said Shang. “We work on this massive amount of raw data, text data from news articles, social media posts, financial reports, scientific papers. ”
While artificial intelligence (AI) can make connections within all this data, creating those models can be labor-intensive, particularly manually annotating data to train a model. Shang and colleagues want to see if they can use existing data to make the process less cumbersome.
“My research focuses on ways to reduce human effort in this model building process,” said Shang. “What's the limit of those machine learning or AI models? How can we teach them with the least amount of effort? For example, can we borrow existing signals from a knowledge base, such as Wikipedia, and generate annotation from this input?”
This approach is called distant supervision, because they are bringing in data from a source intended for a different task. But Shang wants to go a step beyond that into extremely weak supervision, classifying data based on topic, such as sports or politics, or geographic location.
“There's basically no supervision at all,” said Shang. “You only have some seed words or some class names. If we can build a model that accurately classifies documents, based on this small amount of signal, then we are done; we have addressed the problem nicely.”
Early work classifying news articles has performed well, but Shang and colleagues still want to extend this approach into natural language processing and other areas.
Industry vs. Academia
Like many recently minted computer science Ph.D.s, Shang had opportunities in industry as well as academia. He did internships at Google and a hedge fund called Two Sigma. These and others made significant offers, but he opted for UC San Diego.
“At this point I prefer academia because I enjoy working on the problems that interest me, rather than ones that interest the company,” said Shang.
Shang is teaching introductions to data mining and machine learning – high-demand classes that may have 200 students. It’s been a challenge, particularly during the pandemic.
“I try my best to accommodate different levels,” said Shang. “I realized many students have solid backgrounds in math and CS, and they can quickly master everything and they need more advanced work. On the other hand, there are students whose foundations are not as solid, and they really need some hand-holding, especially for their first homework.”
On top of everything, he is coaching the UC San Diego International College Programming Competition (ICPC) team, which has been thriving.
“This year we dominated the Southern California Regional Competition,” he said. “We sent seven teams and one team got first place and another got third place. We significantly outperformed our peers: UCLA, USC, and Cal Caltech.”