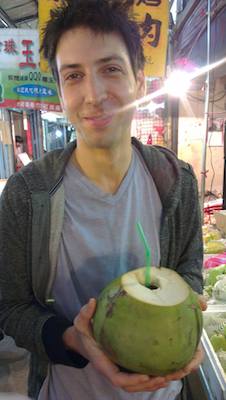
As CSE seeks campus approval for a new major in Data Science and Engineering, Ph.D. student Zachary Chase Lipton asks the question: "Will the real data scientists please stand up?" That's the title of his May 19 column in KDnuggets, which covers data mining, analytics, big data and data scence. In the article, Lipton parses the various definitions of "data scientist," which he says has grown to include "computer scientists, mathematicians, and physicists as well as business school graduates, economists, and other social scientists. Some positions seem to require mathematical maturity, others superior coding skills, and yet more are clearly looking for SQL jockeys, who can generate visualizations and insert them into powerpoint presentations."
Lipton (at right) distills the profession into five archetypes of data scientists. The "theorists" are mostly academics who "primarily study algorithms that are provably efficient and provably correct, even if they must rely on unrealistically strong assumptions," writes Lipton, who works with CSE Prof. Charles Elkan in CSE's Artificial Intelligence group. "Theory papers contain proofs correctness, proofs of convergence, and guarantees on performance." The second archetype is the machine learning scientist, who works in universities or big tech companies such as Google, Amazon, Microsoft and Facebook. Lipton says "machine learning scientists sit somewhere between theorists and data miners," and they develop new algorithms but also "care about empirical performance on real-world tasks." Archetype #3 are the data miners. "These engineers are often strong programmers and combine domain-specific intuition with a knowledge of algorithms to generate valuable insights," writes Lipton, and they work at a broader cross-section of Silicon Valley-type companies as well as in health and other spaces that are focused on mining a particular industry's data.
"Script kiddies" is what Lipton calls the fourth archetypal data scientist, defined as end-users of data science products such as Azure ML, IBM Watson and KNIME. "They may know roughly what a support vector machine does," says Lipton, "but wouldn't code one from scratch." And finally, the loosest definition of data scientist might more appropriately be called "Powerpoint jockeys". They are employed in management consulting firms and elsewhere. They may previously have been called business analysts, but now want a fancier-sounding title such as data scientist. "These individuals may have no coding skills or mathematical background," writes Lipton, "but why should qualifications stand in the way of ambition?" Strong skills in Powerpoint and Excel make it possible to churn out impressive-looking visualizations to justify the "data scientist" moniker.