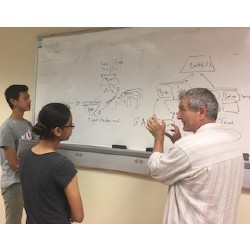
By Doug Ramsey
Can artificial intelligence enable computers to translate a musical composition between musical styles – e.g., from pop to classical or to jazz? According to a professor of music and computer science at UC San Diego and a high school student, they have developed a machine learning tool that does just that.
“People are more familiar with machine learning that can automatically convert an image in one style to another, like when you use filters on Instagram to change an image’s style,” said UC San Diego computer music professor Shlomo Dubnov, who is an affiliate faculty member in the Computer Science and Engineering department. “Past attempts to convert compositions from one musical style to another came up short because they failed to distinguish between style and content.”
To fix that problem, Dubnov and co-author Conan Lu developed ChordGAN – a conditional generative adversarial network (GAN) architecture that uses chroma sampling, which only records a 12-tones note distribution note distribution profile to separate style (musical texture) from content (i.e., tonal or chord changes).
“This explicit distinction of style from content allows the network to consistently learn style features,” noted Lu, a senior from Redmond High School in Redmond, Washington, who began developing the technology in summer 2019 as a participant in UC San Diego’s California Summer School for Mathematics and Science (COSMOS). Lu was part of the COSMOS “Music and Technology” cluster taught by Dubnov, who also directs the Qualcomm Institute’s Center for Research in Entertainment and Learning (CREL).
On July 20, Dubnov and Lu will present their findings in a paper* to the 2nd Conference on AI Music Creativity (AIMC 2021). The virtual conference – on the theme of “Performing [with] Machines” – takes place June 18-22 via Zoom. The conference is organized by the Institute of Electronic Music and Acoustics at the University of Music and Performing Arts in Graz, Austriaia from June 18-22.
After attending the COSMOS program in 2019 at UC San Diego, Lu continued to work remotely with Dubnov through the pandemic to jointly author the paper to be presented at AIMC 2021.
For their paper, Dubnov and Lu developed a data set comprised of a few hundred MIDI audio-data samples from pop, jazz and classical music styles. The MIDI files were pre-processed to turn the audio files into piano roll and chroma formats – training the network to convert a music score.
“One advantage of our tool is its flexibility to accommodate different genres of music,” explained Lu. “ChordGAN only controls the transfer of chroma features, so any tonal music can be given as input to the network to generate a piece in the style of a particular musical style.
To evaluate the tool’s success, Lu used the so-called Tonnetz distance to measure the preservation of content (e.g., chords and harmony) to ensure that converting to a different style would not result in losing content in the process.
“The Tonnetz representation displays harmonic relationships within a piece,” noted Dubnov. “Since the main goal of style transfer in this method is to retain the main harmonies and chords of a piece while changing stylistic elements, the Tonnetz distance provides a useful metric in determining the success of the transfer.”
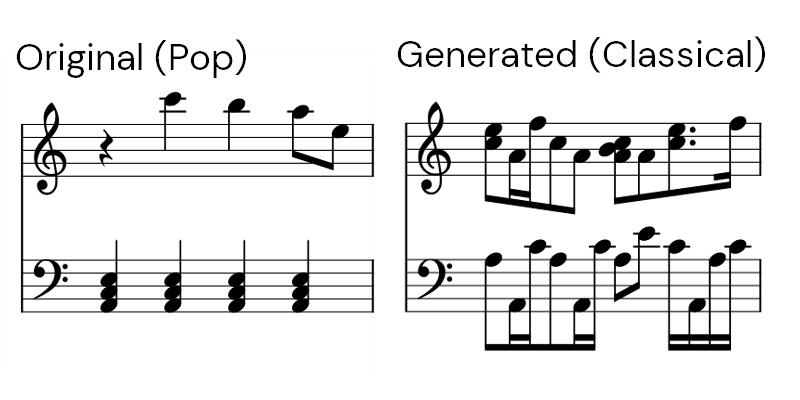
The researchers also added an independent genre classifier (to evaluate that the resulting style transfer was realistic). In testing the accuracy of their genre classifier, it functioned best on jazz clips (74 percent accuracy), and only slightly less well on pop music (68 percent) and classical (64 percent). (While the original evaluation for classical music was limited to Bach preludes, subsequent testing with classical compositions from Haydn and Mozart also proved effective.)
“Given the success of evaluating ChordGAN for style transfer under our two metrics,” said Lu, “our solution can be utilized as a tool for musicians to study compositional techniques and generate music automatically from lead sheets.”
The high school student’s earlier research on machine learning and music earned Lu a bronze grand medal at the 2021 Washington Science and Engineering Fair. That success also qualified him to compete at the Regeneron International Science and Engineering Fair (ISEF), the largest pre-collegiate science fair in the world. Lu became a finalist at ISEF and received an honorable mention from the Association for the Advancement of Artificial Intelligence.
*Lu, C. and Dubnov, S. ChordGAN: Symbolic Music Style Transfer with Chroma Feature Extraction, AIMC 2021, Graz, Austria.