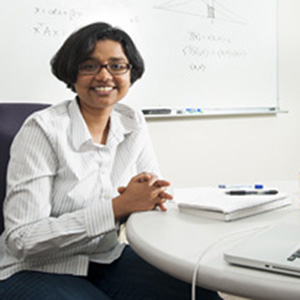
Faculty and students report on research in CSE, ECE, Psychology and Cognitive Science

The 31st Annual Conference on Neural Information Processing Systems (NIPS) took place December 4-9 in Long Beach, CA. NIPS is the largest annual machine learning conference, and this year it attracted nearly 8,000 attendees, including a delegation from Computer Science and Engineering (CSE) and other departments at the University of California San Diego.
“Historically, UC San Diego has always had a strong presence at NIPS,” said CSE professor Kamalika Chaudhuri, who had multiple papers and talks at this year's conference. “Every year we have quite a few papers, and a couple of years ago, the best paper award went to a team from UC San Diego.”
She referred to NIPS 2015, when top honors went to Electrical and Computer Engineering (ECE) professor Alon Orlitsky and his then-Ph.D. student Ananda Theertha Suresh for their paper on “Competitive Distribution Estimation: Why Is Good-Turing Good.” Orlitsky has dual appointments in ECE and CSE, and UC San Diego alumnus Suresh is now a research scientist at Google Research in New York.
NIPS 2017 Tutorial
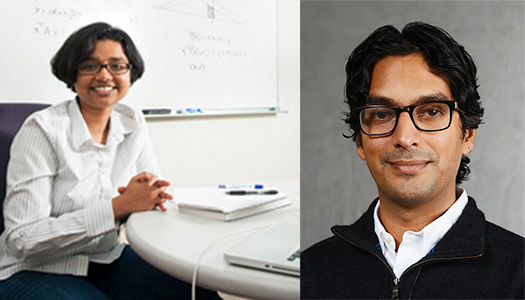
Anand Sarwate, now a professor at Rutgers and
former postdoc at UC San Diego.
UC an Diego’s presence at NIPS 2017 included a tutorial on “Differentially Private Machine Learning: Theory, Algorithms and Applications.” The tutorial was taught by CSE professor Kamalika Chaudhuri and Rutgers University professor Anand Sarwate (who was a postdoctoral researcher in UC San Diego’s Information Theory and Applications Center (ITA) prior to joining the Rutgers faculty). Chaudhuri and Sarwate have posted their NIPS 2017 tutorial slides online. In this tutorial, Chaudhuri and Sarwate described the “basic framework of differential privacy [DP], key mechanisms for guaranteeing privacy, and how to find differentially private approximations to several contemporary machine learning tools,” notably convex optimization, Bayesian methods, and deep learning. Among the final takeaways cited by Chaudhuri and Sarwate: DP measures the risk incurred by algorithms operating on private data; “commonly-used tools in machine learning can be made differentially private; and accounting for total privacy loss can enable more complex private algorithms.”
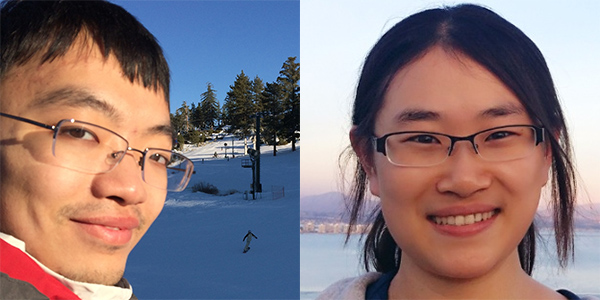
Shuang Song work in the lab of CSE prof.
Kamalika Chaudhuri
Papers with UC San Diego Authors
Professor Chaudhuri was the senior author on two papers accepted to the main research track at NIPS 2017. She co-authored “Renyi Differential Privacy Mechanisms for Posterior Sampling” with CSE Ph.D. students Joseph Geumlek and Shuang Song.
She also co-authored “Approximation and Convergence Properties of Generative Adversarial Learning” with Chaudhuri’s Ph.D. student Shuang Liu and Google’s Olivier Bousquet.
Chaudhuri’s CSE Ph.D. student Songbai Yan collaborated with recent CSE alumnus Chicheng Zhang (Ph.D. ’17) for a paper on “Revisiting Perceptron: Efficient and Label-Optimal Learning of Halfspaces.” Zhang is currently a postdoctoral scholar at Microsoft Research in New York City.
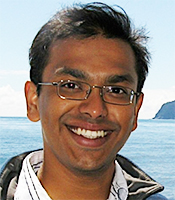
Manmohan Chandraker
collaborated on a paper
with authors from NEC
Labs and the University
of Missouri.
Another CSE professor, Manmohan Chandraker, was senior author on a paper with collaborators from University of Missouri (Guobin Chen and Tony Han) and NEC Laboratories (Wongun Choi and Xiang Yu). Their joint paper was titled “Learning Efficient Object Detection Models with Knowledge Distillation.”
A CSE postdoctoral researcher, Raef Bassily – part of the Data Science Postdoctoral Scholars program in the ITA Center – co-authored a paper on “Practical Locally Private Heavy Hitters” prior to leaving UC San Diego for a faculty position at The Ohio State University. His co-authors on the paper were from Georgetown (Kobbi Nissim), Harvard (Uri Stemmer) and Apple (Abhradeep Thakurta).
Apart from NIPS papers involving faculty or students from CSE, several papers at NIPS 2017 involved faculty and students from another department in the Jacobs School of Engineering.
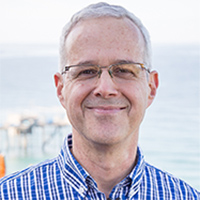
ECE professor Alon Orlitsky has a secondary faculty appointment in the CSE department – and he was the senior author on two papers at this year’s NIPS conference. In both cases, most of his co-authors are Ph.D. students in ECE. His paper on “Maxing and Ranking with Few Assumptions” was co-authored by ECE Ph.D. students Venkatadheeraj Pichapati, Vaishakh Ravindrakumar, Moein Falahalgar and Yi Hao.
Orlitsky also co-authored a paper on “The Power of Absolute Discounting: All-Dimensional Distribution Estimation” with ECE’s Falahatgar and Pichapati along with Mesrob Ohannessian from the Toyota Technological Institute at Chicago.
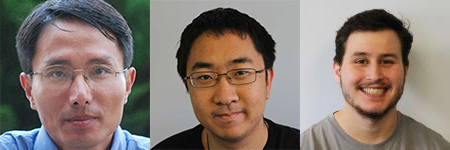
students Long Jin and Justin Lazarow
A Cognitive Science professor, Zhuowen Tu, who also has a faculty appointment in CSE, co-authored a paper with two CSE Ph.D. students, Long Jin and Justin Lazarow (M.S. ’17). Their paper focused on “Introspective Classification with Convolutional Nets.” Professor Tu is also a member of the Jacobs School’s interdisciplinary Center for Visual Computing.
Finally, one paper accepted for presentation in the main track at NIPS 2017 was co-authored by a professor with no connection to CSE or the Jacobs School. Psychology associate professor Edward Vul, who works at the intersection of computational and algorithmic descriptions of human cognition, co-authored a paper on “A Simple Model of Recognition and Recall Memory.” His co-author was IIT Kanpur professor Nisheeth Srivastava, who previously worked as a postdoctoral scholar in the lab of Psychology’s Vul.
Workshops
UC San Diego also had a presence in workshops co-located with NIPS 2017. Professor Chaudhuri also delivered two invited talks at NIPS 2017 co-located workshops. One was delivered at the Dec. 8 day-long Workshop on Nearest Neighbors for Modern Applications with Massive Data. Her talk, based on a joint paper with third-year CSE Ph.D. student Yizhen Wang and Somesh Jha from the University of Wisconsin-Madison, focused on “Analyzing Robustness of Nearest Neighbors to Adversarial Examples.” According to Chaudhuri, there is an overall lack of general understanding about the foundations of designing machine learning algorithms robust to adversarial examples. “We take a step towards addressing this challenging question by introducing a new theoretical framework, analogous to bias-variance theory, which we can use to tease out the causes of vulnerability,” she noted. “We apply our framework to a simple classification algorithm: nearest neighbors, and analyze its robustness to adversarial examples.” Chaudhuri also proposed a modified version of the nearest neighbor algorithm, and demonstrated both theoretically and empirically that it has superior robustness to standard nearest neighbors.
Chaudhuri also delivered an invited talk on "Privacy-Preserving Mechanisms for Correlated Data," which was part of the Machine Learning and Computer Security Workshop (also co-located with NIPS 2017). It was one of three invited talks in the session on formal definitions and formal verification.
At another workshop, CSE Ph.D. student Songbai Yan collaborated with his co-advisors, CSE’s Chaudhuri and Electrical and Computer Engineering (ECE) professor Tara Javidi on a joint paper on, "Active Learning with Logged Bandit Feedback", where instances are drawn from an underlying distribution, and a logging policy is used to determine whether they should be assigned a binary label. The talk was part of the From What-If to What-Next Workshop. “Our goal is to learn a binary classifier that predicts labels with high accuracy on the entire population, not just the distribution of the logged data,” according to the paper’s abstract. “Previous work addresses this problem either when only logged data is available, or purely in a randomized experimentation setting.” The authors combined both approaches to provide an algorithm that uses logged data to bootstrap and inform experimentation, thus achieving the best of both worlds.