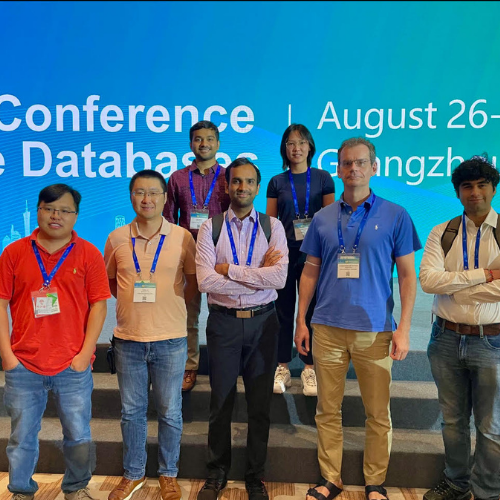
A decade ago, machine learning and artificial intelligence were greeted with skepticism by the database community. The topics were largely dismissed. But fast forward to 2024 where the sentiment has changed.
The 50th International Conference on Very Large Databases (VLDB), held this summer in Guangzhou, China, saw an explosion of interest at the intersection of ML/AI and data management and data systems. With multiple dedicated research sessions, panels, and workshops focused on this sub-area, it was the leading topic at the forum, eclipsing over a dozen others.
Computer scientists from the University of California, San Diego were at the forefront of these ML/AI-related discussions at VLDB 2024. The cohort of thought leaders included Arun Kumar, an associate professor in the Jacobs School of Engineering’s Department of Computer Science and Engineering (CSE) and the Halıcıoğlu Data Science Institute (HDSI), along with former CSE Professor and now Adjunct Professor Yannis Papakonstantinou, and three CSE alumni.
“When I started as assistant professor in 2016, most folks believed deep learning and AI were hype that would fizzle out. At VLDB back then, I saw only a handful of papers focused on data management and systems problems in AI,” said Kumar.
According to Kumar, less than 5 percent of papers published at the VLDB conference eight years ago were related to ML/AI. By comparison, this intersectional sub-area represented almost 25 percent of published papers this year. Kumar attributes the growth to a recent “boom” fueled by Large Language Models (LLMs).
The center of this boom is occupied by Kumar’s own extensive research. Kumar was recognized at the conference with the VLDB Early Career Research Contribution Award for his impact in the field. He also spoke on a panel discussion titled, "Data Science Challenges and Opportunities in the LLM Era," exploring the far-reaching implications of LLMs on data science.
“My work makes deep learning systems substantially faster, cheaper, and easier to use by reimagining their execution stack with inspiration from decades of work in the data systems world,” said Kumar.
Specifically, Kumar’s award-winning research draws analogies to techniques known as "multi-query optimization" in the database systems world and proposes a suite of novel system design and optimization techniques for deep learning workloads. As a bonus, they are easy to adopt.
Consequently, Kumar’s ideas are democratizing AI beyond big tech firms. His work has been adopted in the domain sciences at UC San Diego, by industry collaborators, and by a VC-backed software startup Kumar co-founded named RapidFire AI.
“The boom is not just in the research world, but also in the database systems and cloud computing industries,” said Kumar. “As per the International Data Corporation, the market for products to make it easier for organizations to adopt modern AI, including new applications by LLMs, will represent almost half a trillion a year market size by the end of this decade.”
Two of Kumar’s former PhD students, who co-authored conference papers with him, presented their work at VLDB 2024. Kabir Nagrecha (BS ’21, PhD ’24) presented the paper, SATURN: An Optimized Data System for Multi-Large-Model Deep Learning Workloads. The study proposes a new information system architecture, dubbed SATURN, designed to resolve three training burdens for large models: model selection, resource apportioning, and scheduling. Ideas from this work are being adopted by Netflix and Meta for their AI infrastructure, as well as by Kumar’s own startup.
CSE alumnus Vraj Shah (PhD ’22), currently a staff research scientist at IBM Research, presented the paper, How do Categorical Duplicates Affect ML? A New Benchmark and Empirical Analyses. Shah and his co-authors, including Kumar, offered the first systematic scientific study to analyze the impact of Categorical duplicates on ML and presented novel data artifacts, benchmarks, and empirical analyses to guide future research.
In another close tie to the university, Papakonstantinou, who shares his time with Google Cloud, participated in a panel discussion on the topic, “Vector Databases: What’s Really New and What’s Next?” Moderated by Papakonstantinou’s former student Jianguo Wang (PhD ’18), now an assistant professor at Purdue University, the session provided insights on the future of vector databases and the broader role of databases in the era of generative AI.
VLDB is a premier annual international forum for data management, scalable data science and database researchers, vendors, practitioners, application developers, and users. It covers issues in data management, database architectures, graph data management, data privacy and security, data mining, machine learning, AI, and database systems research.
--By Kimberley Clementi